All
Latest News
Publications
Machine learning can help in assessing the efficacy of using the insect growth regulator pyriproxyfen on insecticide-treated nets
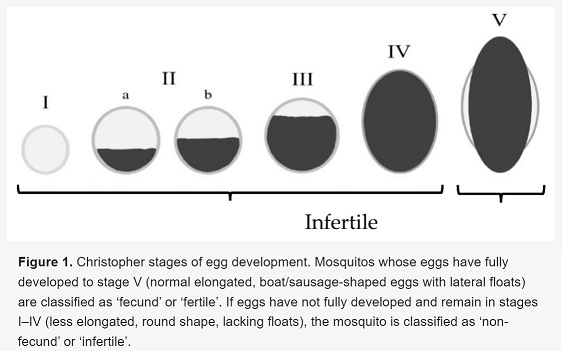
A new method for measuring the effect of pyriproxyfen on mosquito fertility using a machine learning approach has been published
In order to fight the threat posed to malaria control by the spread of pyrethroid resistance in mosquitoes, we need insecticide treated nets (ITNs) with novel active ingredients against these insects. Insect growth regulators like pyriproxyfen (PPF) show great promise in areas where the majority of mosquitoes are pyrethroid-resistant. PPF negatively affects the reproductive output of female mosquitoes by reducing their fertility and lifetime fecundity, in addition to their longevity. In essence, it can greatly reduce the progeny of these vectors and so their populations. A recent study showed that PPF-treated ITNs effectively sterilize females of Anopheles gambiae (the major malaria vector in Africa) under both laboratory and field conditions.
But how can we measure the efficacy and durability of PPF-treated nets or other vector control tools? Since exposure to PPF inhibits development of mosquito ovaries, assessing their appearance is a tried and tested method. However, this approach requires expertise that is difficult to train and is not available in many contexts. There is then a need for making the classification of ovary development more accessible and ideally automated.
The use of artificial intelligence (AI) and machine learning can be a great asset in this context, as shown in the recent work of I2I members published as part of the special issue on mosquito control in the journal Insects. Using a Convolutional Neural Network (CNN) model, researchers were able to automatically classify the fertility status of ovaries from PPF-exposed female mosquitoes. The CNN was “trained” to recognize important features in dissected ovaries using a large dataset of images from mosquitoes whose PPF exposure and fertility status were already determined. When tested, the model was able to correctly classify the ovaries with 94% accuracy with an average time of less than 40 seconds.
Dr. Mark Fowler, lead author and Data Manager in the Vector Biology department at LSTM, gave insight into the importance of this tool: “A model of this kind could provide an effective and free means for monitoring the efficacy and durability of PPF-treated tools in settings were the expertise to assess ovary development is not easily available.”
Access to the publication can be obtained at: https://www.mdpi.com/2075-4450/12/12/1134
References:
Grisales N, Lees RS, Maas J, Morgan JC, Wangrawa DW, Guelbeogo WM, N’Fale S, Lindsay SW, McCall PJ, Ranson H. Pyriproxyfen-treated bed nets reduce reproductive fitness and longevity of pyrethroid-resistant Anopheles gambiae under laboratory and field conditions. Malar J. 2021 Jun 22;20(1):273. doi: 10.1186/s12936-021-03794-z.